Electric Vehicle Conference 2016
Topic: Electric Vehicle Conference 2016
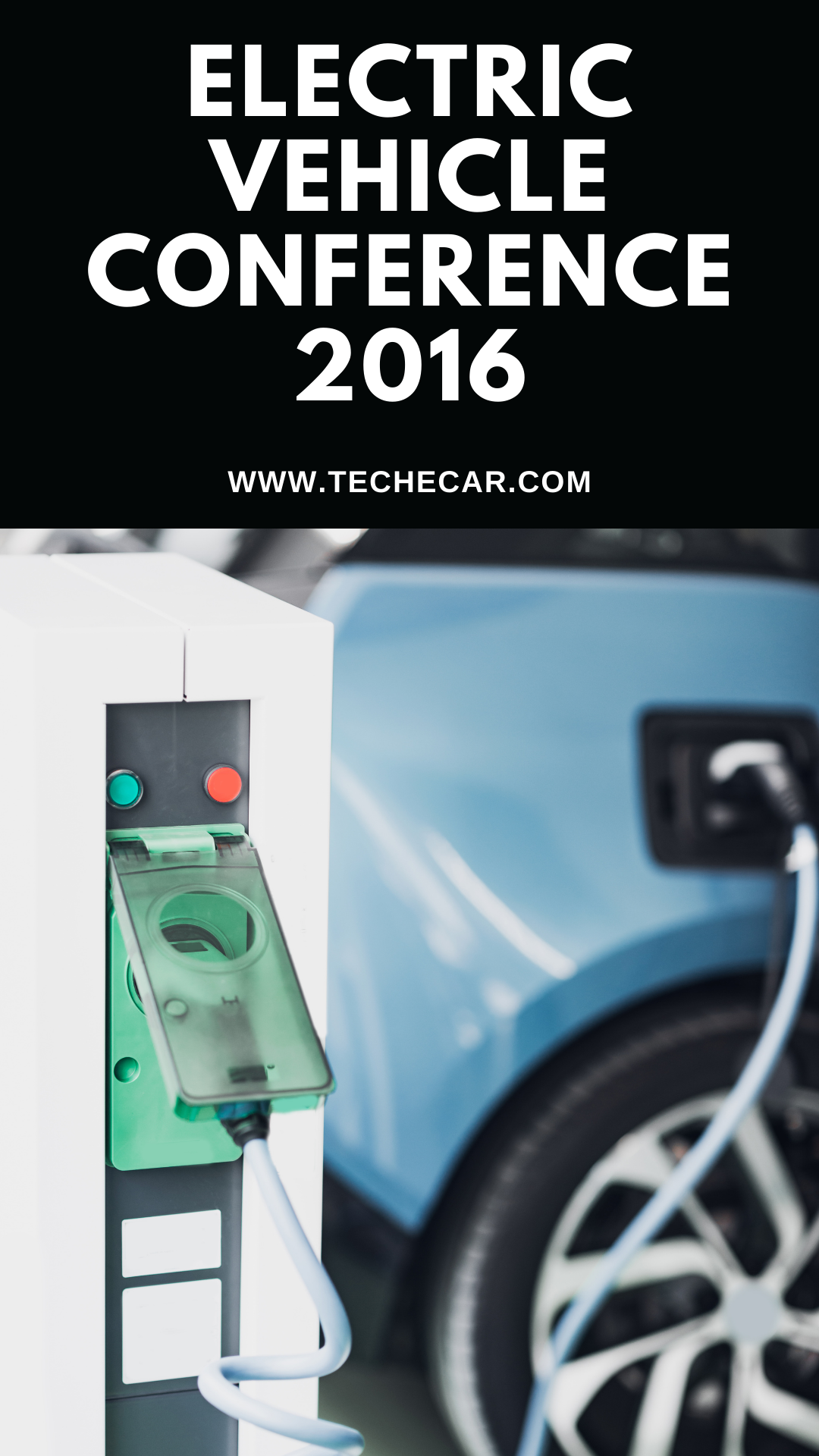
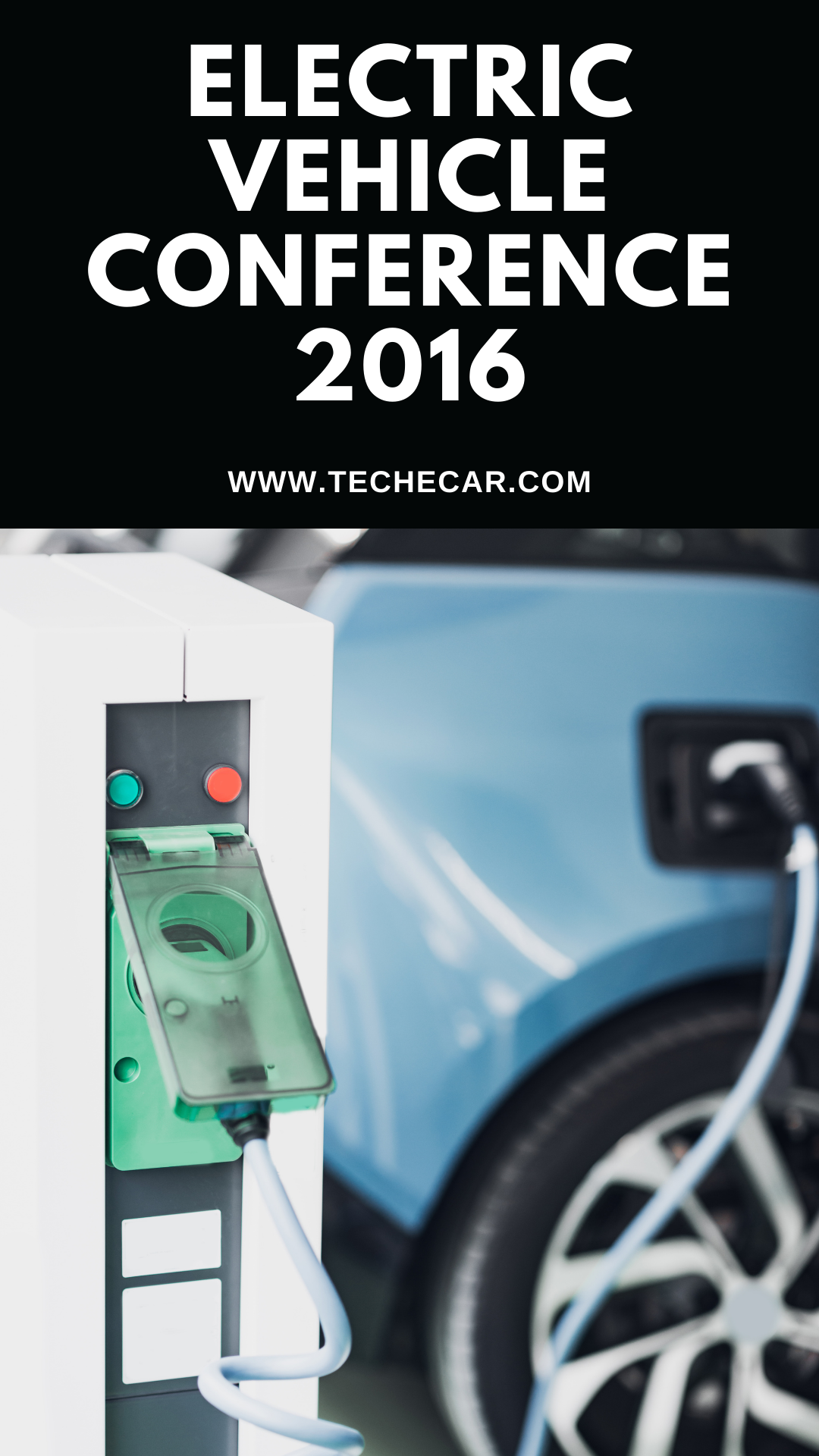
Electric Vehicle Conference 2016 ABSTRACT
Abstract
Greenhouse gas emissions could be reduced by using electric automobiles. Research on electric vehicles and their charging infrastructure has been considerable; nonetheless, there is still a lot of room for improvement in the development and network modelling of electric vehicles.
A comprehensive assessment of electric vehicle studies is presented in this article, which identifies existing research gaps in theories, modelling techniques, solution algorithms, and applications.
Electric car concepts, market share, attributes and charging infrastructures are initially discussed in this article. Studies on the traffic assignment problem for electric vehicles on a network with limited recharge stations are also examined.
It is critical to consider the unique properties of electric vehicles (such as their range limit) while anticipating their routing patterns and designing charging infrastructure networks.
Electric Vehicle Conference 2016 INTRODUCTION
Introduction
Since the Kyoto Protocol was signed in 1998, governments worldwide have been working to address the serious problem of carbon-based emissions and greenhouse gases (GHGs). Oil, which accounts for 98 per cent of transportation’s energy needs, is heavily influenced by variations in energy supply.
The electric vehicles’ market has been supported by government and auto industry initiatives that acknowledge the need for alternative fuel vehicles (AFVs) for environmentally-friendly mobility.
One AFV that can minimise GHG emissions is the plug-in hybrid electric vehicle (PHEV). The future of hybrid gasoline-electric vehicles is bright since they can lower gasoline consumption and GHG emissions by 30 per cent to 50 per cent without changing the vehicle class.
Although electric vehicles can travel between 150 and 200 kilometres on a single charge, the limited battery capacity prevents them from becoming more widely used.
While the chicken and egg issue persists, the most intractable hurdle is manufacturing and acquiring these vehicles and creating the refuelling infrastructure before these vehicles are built.
Battery electric vehicle (BEV) drivers’ travel habits will be restricted to some degree because of the limited availability of recharging infrastructure in the not-too-distant future.
When plug-in electric vehicles (PEVs) become common, existing network flow modelling tools will have to undergo fundamental changes to account for the new behaviours that may emerge and the resulting constraints on demand forecasting and the evaluation of transportation development plans.
EV network modelling is the focus of this article, covering a wide range of subjects. It’s important to note that this piece has the following structure and primary focus:
- The research on charging infrastructure form and area are briefly described under “Required to charge stations style and placement studies.” EV market growth and acceptability hinge heavily on these factors.
- ‘PEV market potential, demand and behaviour study’ examines the market potential and demand for PEVs in terms of EV infrastructure, difficulties and prospects. There are then comparisons between electric vehicles and regular gasoline-powered automobiles in terms of attributes and driving habits.
- The report presents the TAP of vehicles with a restricted range in section ‘TAP of EVs with limited charging infrastructure. Additional constraints, like driving range and charging availability, need to be taken into account as the EV SPP, which is a subproblem of TAP.
- The logistic issue of the EV vehicle routing problem (VRP), which generalises the well-known travelling salesman problem and commonly uses distance or energy-constrained SPP as its subroutine, is explained here.VRP
- Section ‘Network design and bi-level model’ discusses the EV’s network design issue (NDP) and the bi-level models used to solve the TAP. There are two levels to the bi-level model: the upper level, where charging facilities are located, and TAP.
To conclude, the research gaps in network modelling of electric vehicles have been identified and proposed research directions.
Electric Vehicle Conference 2016- Design and location studies for charging stations
Design and location studies for charging stations
Electric vehicle drivers require charging stations. Because of the high expense of creating these facilities, suppliers such as EV firms and governments are worried about where to install charging stations and what sort of charging station to locate.
Although many cities intend to build and expand charging infrastructure for BEVs, BEV commuters will likely need to charge their vehicles at home the majority of the time shortly.
The current way of charging the vehicle battery in many EVs, such as the Nissan Leaf or Chevrolet Volt, is to plug the battery into the power grid at home or work. The battery takes a long time to recharge, limiting EVs’ use to short-distance trips.
EV manufacturers are attempting to solve this limited range requirement by installing fast-charging stations that can charge a vehicle in a matter of minutes.
Bai et al. proposed an optimal design of a rapid charging station for PHEVs and EVs to reduce power grid pressure while providing vehicles with the necessary power.
Qiu et al. used the stochastic service system and queuing theory to optimise the allocation number of EV chargers by analysing the characteristics of EVs’ arrival time and charging duration in fast-charging stations.
Compared to gasoline vehicles, EVs require more time to recharge, and the fast charging station is more expensive to maintain. These inherent issues, combined with a lack of charging infrastructure, severely limit the widespread adoption of EVs.
These issues are more noticeable on longer travels, such as intercity trips. Range anxiety (the fear that the car will run out of charge before arriving at the destination) is a key impediment to the market penetration of EVs.
Hybrid vehicles, which contain an electric motor and a gasoline engine, can help to ease range anxiety to some extent. However, because hybrids still require gasoline, these vehicles do not alleviate the environmental consequences.
Another type of refuelling infrastructure is fast battery exchange stations (BESs) or BSSs. These stations will take a pallet of nearly expended batteries from a vehicle and replace it with a battery pallet already charged.
This method of refuelling has the advantage of being quite quick. The terrible drawback is that all of the cars served by the BES must use the same pallets and batteries.
It is believed that the developers of these battery pallets will band together to create a single universal standard. In 2010, taxi vehicles in Tokyo experimented using BESs. Denmark is looking at the idea of having enough battery interchange stations so that the country can rely on no, or very few, gasoline-powered automobiles.
There is a geographical issue regarding where to site these refuelling stations, including battery recharging, battery exchanging, and various alternative refuelling choices. Several scholars have explored optimally situating such refuelling stations using the flow refuelling placement model.
Models of placing the fewest charging stations on the shortest road are proposed to enable EV mobility to eliminate charging diversions. A conceptual optimisation model is proposed to analyse EV travel throughout a long corridor.
The goal is to choose the battery size and charge capacity (in terms of charging power at each station and the number of stations required along the corridor) to fulfil a specified level of service while minimising the total social cost.
Wang and colleagues suggested a refuelling station location model based on vehicle routing logic and a set cover concept that takes both inter-city and intra-city travel into account.
MirHassani and Ebrazi established a flexible mixed-integer linear programming approach by reformulating the flow refuelling location model. The model can solve the top cover form and obtain an optimal solution faster than the prior set cover version.
Xi et al. used a simulation–optimisation model to decide where to place EV chargers to maximise their utilisation by privately owned EVs. Dong et al. investigated EV charging station location issues and the influence of public charging station deployment on increasing the travel kilometres of EVs.
Electric Vehicle Conference 2016- Study of the potential, demand, and behaviour of the PEV market
Study of the potential, demand, and behaviour of the PEV market
In terms of PEV market potential, the future automobile is the hybrid gasoline–EV, which will most likely become the dominant vehicle platform by 2020. GPS data from the houses were used to demonstrate how PEVs can meet the needs of different households (single-vehicle or multiple-vehicle).
Cost comparisons were made between the PHEV and a typical gasoline vehicle, and annual savings were calculated. The reduction in transportation costs and GHG emissions provided by a PHEV compared to a comparable conventional vehicle was also highlighted.
Smart and Schey examined the Nissan Leaf, a BEV, and discovered that drivers averaged 6.9 miles per trip, 30.3 miles per day, and 1.05 charging events per day, with 82 per cent of charging events taking place at home.
Chargers and cables are classified according to voltage and power levels: Level I is 120 V alternating current (AC) up to 20 A (2.4 kW); Level II is 240 V AC up to 80 A (19.2 kW); and Level III, which has yet to be completely defined, will likely be 240 V AC and larger at power levels of 20–250 kW.
The SAE J177232 standard specifies a five-pin layout for Level I and Level II charging. A Level III connector is being developed, as is the usage of the current connector for direct current (DC) power flow.
Markel described the components of the PEV infrastructure, the obstacles and opportunities associated with the infrastructure’s design and deployment, and the possible benefits.
Dong et al. used longitudinal travel data to offer a stochastic modelling strategy for characterising BEV drivers’ behaviour. It takes into account a more realistic examination of the influence of charging stations on BEV practicality.
The actual range of a BEV is expressed as a Weibull-distributed variable, but the between-charge travel distances are expressed as a Poisson–gamma distribution.
Hide et al. used a stated preference study to examine customers’ willingness to pay for EVs and their features. It revealed that driving range, fuel cost reductions, and charging time are the most critical considerations and that battery costs must fall dramatically before EVs can enter the mainstream market without subsidies.
He et al. devised a model that incorporates the interplay between public charging possibilities, power pricing, and PHEV destination and route choices.
TAP for vehicles with a limited range
Traffic assignment is generally defined as an uncapacitated nonlinear multi-commodity network flow issue with an optimum or equilibrium routing principle. It is the fourth and last phase in the classic four-step travel demand modelling procedure.
It is frequently used as an evaluation tool for various analyses of urban and regional traffic networks.
The conventional TAP can be effectively solved using a Frank–Wolfe type algorithm, with the linearised sub-problem of finding the shortest pathways between each O–D pair.
Ichimura et al. first presented the difficulty of finding the shortest path for an EV. A vehicle has a limited battery and can stop and recharge at specific sites. Lawler devised a polynomial algorithm to solve EV SPP, making it polynomially solvable.
Several studies addressed EVs in traffic assignment; however, they limited EV travel distances and assumed no refuelling. Adding refuelling stations to the shortest weight-constrained route problem (SWCPP) is an NP-complete problem that Laporte and Pascoal have studied.
EV VRP, EV SPPs with various EV special limitations are thoroughly investigated. These can be put into TAP as EV network subroutines to expand the TAP with the EV network family.
Numerous publications have been published that handle the classical VRP with capacity and distance limits. Erdogan and Miller updated the VRP to account for the different problems of operating an AFV fleet, such as the driving range limit and local refuelling infrastructure.
Adler et al. suggested an EV shortest-walk issue to find the shortest trip distance route that includes cycles for detouring to recharging batteries from origins to destinations with the least amount of detours.
Cabral et al. investigated the undirected graph network design problem with relays (NDPR), which generalised the SPP with relays and the weight-constrained SPP. The distance between two consecutive relays does not exceed a predetermined upper limit.
The problem of energy-efficient routing of EVs has been addressed, and polynomial-time algorithms have been developed in the literature by taking into account the EV’s limited cruising range and regenerative braking (i.e. the EV increases its level of energy when braking), which is a special case of the constrained SPP. Ryan and Miguel created a recharging VRP that allows vehicles with a restricted range to recharge at client locations.
A substantial amount of study on optimal EV route planning is presented. Other difficulties concerning the battery-swapping service have also been considered within other frameworks.
From the standpoint of operational research, Mirchandani et al. presented several new logistics pertinent to designing and operating a fleet of EV vehicles operating within a battery-exchanging infrastructure.
Furthermore, Jiang et al. developed a network equilibrium issue with integrated destination, route, and parking options constrained by the driving range limit and alternate travel cost composition associated with BEVs.
Electric Vehicle Conference 2016- Design of a network and a bi-level model
Design of a network and a bi-level model
The NDP is concerned with altering a transportation system, such as adding new links or improving existing ones, to reduce total system costs, including system travel costs and investment costs.
It involves situating EV charging stations in the traffic network and minimising the cost of charging station investment and trip cost in the EV scheme. This equilibrium NDP can be expressed using the bi-level programming approach.
Wang et al. created a global optimisation method for a discrete NDP that may be used in the design of EV networks. It is expressed as a bi-level programming model, with the top-level aiming to minimise overall cost (sum of total journey times and investment costs).
The lower level is a standard Wardrop’s user equilibrium (UE) problem. The bi-level model has been used in several congestion pricing schemes to construct the toll for the transportation network. These models and solution algorithms can be adapted to the EV scheme, given the identical framework and limitations.
Developments in the future
Even though there is substantial literature on the EV scheme, the EV network modelling study is restricted and evolving, including the EVs’ limited driving range, various charging facilities, and a lack of charging facilities.
There have been few studies on the stochastic or dynamic traffic assignment of EVs and those that take into account elastic demand. At the moment, the two fundamental characteristics of EVs are their limited driving range and a lack of charging infrastructure.
To the best of our knowledge, the problem of developing the universal stochastic user equilibrium (SUE) traffic assignment model or dynamic traffic assignment model with path distance constraints, as well as the accompanying solution techniques, remains unsolved.
Consider the stochastic traffic assignment model. It turns out that adding path distance constraints to Daganzo’s model, which is an unconstrained minimisation model, does not optimise one of the modified SUE conditions.
Electric Vehicle Conference 2016- Algorithms for stochastic traffic
Algorithms for stochastic traffic assignment with path distance restrictions for large-scale networks that are efficient, concurrent, and resilient
The objective function of the SUE model with path-specific restrictions contains path-specific terms that lack explicit mathematical formulations, making the final model more complex to solve the global optimality than traditional traffic assignment models.
Furthermore, existing stochastic traffic assignments with side restrictions solution techniques have only been applied to small networks. The algorithms’ resilience has not yet been tested.
To achieve sufficient execution time and accuracy for probit-based SUE problems on the large-scale network, a distributed computing system could be of significant importance. As a result, establishing globally consistent and effective methods for huge EV transportation systems is a difficult future study subject.
The impact of EV signal priority on microscopic EV behaviour
Signal priority is an efficient method of reducing vehicle delay at signalised junctions. Many cities worldwide have taken initiatives to increase the market share of electric vehicles, such as government subsidies, free parking, and signal priority.
However, the prospective impact of the measures mentioned above is unknown. Furthermore, car following, overtaking, and lane changing may differ amongst EV classes.
A car-following model has been presented to investigate the effects of EV driving range on driving behaviour. Consider a link with a variable number of lanes.
The number of lanes has been observed to alter platoon dispersion of traffic flow in low-friction circumstances. As a result, specific tiny EV behaviours remain a promising future direction.
Electric Vehicle Conference 2016- Potential consequences
Potential consequences when qualities associated with EV change
Recent technological advancements show that driving range can be increased, charging time can be reduced, and battery costs can be reduced. Furthermore, as with most new technologies, the unit cost of EVs is expected to reduce after a few years of mass manufacturing.
Attributes have a significant impact on different elements of EV adoption. Oil and electricity costs will impact fuel cost savings while charging time and driving range will cause range anxiety. As a result, it is critical to comprehend the market for EVs in the future when there is a strong push and significant investment in EVs.
Location of charging stations on urban highways
Urban highways have been identified as critical transportation infrastructure. Recent charging station location research has been focused on metropolitan areas like the Central Business District (CBD), with typical p-median, p-centre, maximum covering, flow capturing, and flow interception models employed to maximise coverage.
Given the driving range of EVs, installing charging stations on metropolitan freeways is increasingly vital and encouraged to alleviate range anxiety. As a result, there is a pressing need to establish an ideal charging station location model on metropolitan freeways, particularly for intercity trips.
PDF LINKS FOR Electric Vehicle Conference 2016
Powertrain development for electric vehicles: trial effective program evaluation
Registration Fees for 2016 Plug-In Hybrid and Electric Vehicles
Electric Vehicle Feasibility
Current electric car research
29th International Electric Vehicle Symposium 2016 (EVS29)
Recommended Articles: